If you’re a software engineer preparing for a machine learning (ML) interview at Amazon, you’re probably feeling a mix of excitement and nerves. Amazon is one of the most innovative companies in the world, and its ML teams are at the forefront of cutting-edge technologies like Alexa, AWS, and recommendation systems. But with great innovation comes a rigorous interview process.
In this blog, we’ll break down the top 25 frequently asked questions in Amazon ML interviews and provide detailed answers to help you prepare. Whether you’re a seasoned ML engineer or just starting out, this guide will give you the confidence to ace your interview. And hey, if you need extra help, InterviewNode (that’s us!) is here to support you every step of the way.
Let’s get started!
1. Introduction: Why Amazon ML Interviews Are a Big Deal
Amazon is a global leader in machine learning and artificial intelligence. From personalized product recommendations to Alexa’s voice recognition, ML is at the heart of Amazon’s success. As a result, the company looks for top-tier talent who can not only understand complex ML concepts but also apply them to solve real-world problems at scale.
But here’s the thing: Amazon’s ML interviews are tough. They test your technical skills, problem-solving abilities, and alignment with Amazon’s Leadership Principles. The good news? With the right preparation, you can crack the code and land your dream job.
In this blog, we’ll cover:
-
The structure of Amazon’s ML interview process.
-
The top 25 questions you’re likely to face, along with detailed answers.
-
Tips to stand out during the interview.
-
How InterviewNode can help you prepare effectively.
Ready? Let’s dive in!
2. Overview of Amazon’s Machine Learning Interview Process
Before we jump into the questions, let’s understand what the interview process looks like. Amazon’s ML interviews typically consist of the following stages:
1. Screening Round
-
A recruiter or hiring manager will assess your resume and experience.
-
You may be asked to complete an online assessment or coding challenge.
2. Technical Rounds
-
ML Fundamentals: Questions on supervised/unsupervised learning, overfitting, bias-variance tradeoff, etc.
-
Coding and Algorithms: Implementing ML algorithms, optimizing code, and solving data-related problems.
-
System Design: Designing scalable ML systems, data pipelines, and model deployment strategies.
3. Behavioral and Cultural Fit
-
Amazon places a strong emphasis on its Leadership Principles. Be prepared to answer questions like, “Tell me about a time you disagreed with a teammate” or “How do you prioritize tasks when faced with tight deadlines?”
4. Onsite Interviews
-
A series of in-depth technical and behavioral interviews, often conducted in person or via video call.
Now that you know what to expect, let’s tackle the top 25 questions you’re likely to face.
3. Top 25 Questions
Section 1: Machine Learning Fundamentals
1. What is the difference between supervised and unsupervised learning?
-
Supervised Learning:
-
The model is trained on labeled data, where each input has a corresponding output.
-
Examples: Predicting house prices (regression), classifying emails as spam or not spam (classification).
-
Algorithms: Linear regression, logistic regression, support vector machines (SVM), neural networks.
-
Use Case: When you have a clear target variable and want to predict outcomes based on input features.
-
-
Unsupervised Learning:
-
The model is trained on unlabeled data and must find patterns or structures on its own.
-
Examples: Grouping customers into segments (clustering), reducing the dimensionality of data (PCA).
-
Algorithms: K-means clustering, hierarchical clustering, principal component analysis (PCA).
-
Use Case: When you want to explore the data and uncover hidden patterns without predefined labels.
-
Pro Tip: In real-world applications, semi-supervised learning (a mix of labeled and unlabeled data) is often used to leverage the benefits of both approaches.
2. How do you handle overfitting in a machine learning model?
Overfitting occurs when a model learns the training data too well, including noise and outliers, and performs poorly on unseen data. Here’s how to handle it:
-
Cross-Validation: Use techniques like k-fold cross-validation to evaluate the model’s performance on multiple subsets of the data.
-
Regularization: Add penalty terms to the loss function to discourage complex models.
-
L1 regularization (Lasso): Encourages sparsity by adding the absolute value of coefficients.
-
L2 regularization (Ridge): Adds the squared value of coefficients to prevent large weights.
-
-
Simplify the Model: Reduce the number of features or use techniques like pruning for decision trees.
-
Increase Training Data: More data helps the model generalize better.
-
Early Stopping: Stop training when the validation error starts to increase (common in neural networks).
Example: If you’re training a neural network and notice the training accuracy is 99% but the validation accuracy is 70%, you’re likely overfitting. Try adding dropout layers or reducing the number of neurons.
3. Explain the bias-variance tradeoff.
-
Bias: Errors due to overly simplistic assumptions in the model. High bias causes underfitting, where the model fails to capture the underlying patterns in the data.
-
Example: Using a linear model to fit non-linear data.
-
-
Variance: Errors due to the model’s sensitivity to small fluctuations in the training set. High variance causes overfitting, where the model captures noise instead of the signal.
-
Example: A decision tree with too many branches.
-
Balancing the Tradeoff:
-
Increase model complexity to reduce bias (e.g., add more layers to a neural network).
-
Simplify the model to reduce variance (e.g., use regularization or reduce the number of features).
Pro Tip: Use learning curves to visualize bias and variance. If the training and validation errors are both high, the model has high bias. If the training error is low but the validation error is high, the model has high variance.
4. What is cross-validation, and why is it important?
Cross-validation is a technique to evaluate a model’s performance by splitting the data into multiple subsets and training/testing on different combinations. The most common method is k-fold cross-validation:
-
Split the data into k subsets (folds).
-
Train the model on k-1 folds and validate it on the remaining fold.
-
Repeat this process k times, using a different fold for validation each time.
-
Average the results to get the final performance metric.
Why It’s Important:
-
Provides a more accurate estimate of the model’s performance on unseen data.
-
Reduces the risk of overfitting by ensuring the model is tested on different subsets of the data.
Example: If you’re working with a small dataset, 10-fold cross-validation can help you make the most of the available data.
5. How do you evaluate the performance of a classification model?
-
Accuracy: The percentage of correctly classified instances. Suitable for balanced datasets.
-
Formula: (True Positives + True Negatives) / Total Instances.
-
-
Precision: The percentage of positive predictions that are correct. Important when false positives are costly.
-
Formula: True Positives / (True Positives + False Positives).
-
-
Recall: The percentage of actual positives correctly identified. Important when false negatives are costly.
-
Formula: True Positives / (True Positives + False Negatives).
-
-
F1-Score: The harmonic mean of precision and recall. Useful for imbalanced datasets.
-
Formula: 2 (Precision Recall) / (Precision + Recall).
-
-
ROC-AUC: Measures the model’s ability to distinguish between classes. A value of 1 indicates perfect classification.
Example: In a fraud detection system, recall is more important than precision because you want to catch as many fraudulent transactions as possible, even if it means some false positives.
Section 2: Algorithms and Models
6. How does a decision tree work?
A decision tree is a tree-like model where each internal node represents a decision based on a feature, each branch represents an outcome of the decision, and each leaf node represents a class label or a continuous value.
How It Works:
-
Start with the entire dataset at the root node.
-
Split the data into subsets based on the feature that provides the best split (using criteria like Gini impurity or information gain).
-
Repeat the process for each subset until a stopping condition is met (e.g., maximum depth or minimum samples per leaf).
Example: Predicting whether a customer will buy a product based on age, income, and browsing history.
Pro Tip: Decision trees are prone to overfitting. Use techniques like pruning or ensemble methods (e.g., random forests) to improve performance.
7. What is the difference between random forests and gradient boosting?
-
Random Forests:
-
An ensemble method that builds multiple decision trees independently and averages their predictions.
-
Reduces variance and avoids overfitting by introducing randomness (e.g., random subsets of features).
-
Suitable for high-dimensional data and robust to outliers.
-
-
Gradient Boosting:
-
An ensemble method that builds trees sequentially, with each tree correcting the errors of the previous one.
-
Focuses on reducing bias and often achieves higher accuracy than random forests.
-
Requires careful tuning of hyperparameters like learning rate and tree depth.
-
Example: Use random forests for quick, robust models and gradient boosting for high-performance models with more tuning.
8. Explain the concept of regularization in machine learning.
Regularization is a technique used to prevent overfitting by adding a penalty term to the loss function. This penalty discourages the model from learning overly complex patterns that may not generalize well to unseen data.
Types of Regularization:
-
L1 Regularization (Lasso):
-
Adds the absolute value of the coefficients to the loss function.
-
Encourages sparsity, meaning some coefficients can become exactly zero.
-
Useful for feature selection when you have many irrelevant features.
-
Formula: Loss = Original Loss + λ * Σ|weights|.
-
-
L2 Regularization (Ridge):
-
Adds the squared value of the coefficients to the loss function.
-
Encourages small weights but doesn’t force them to zero.
-
Useful when all features are relevant but need to be controlled.
-
Formula: Loss = Original Loss + λ * Σ(weights²).
-
-
Elastic Net:
-
Combines L1 and L2 regularization.
-
Useful when you have correlated features and want to balance sparsity and weight shrinkage.
-
Example: In linear regression, adding L2 regularization (Ridge) can help reduce the impact of multicollinearity (high correlation between features).
Pro Tip: The regularization parameter (λ) controls the strength of the penalty. Use cross-validation to find the optimal value of λ.
9. How does a neural network learn?
A neural network learns by adjusting the weights of connections between neurons to minimize the loss function. Here’s a step-by-step breakdown:
-
Forward Propagation:
-
Input data is passed through the network, layer by layer, to produce an output.
-
Each neuron applies a weighted sum of inputs followed by an activation function (e.g., ReLU, sigmoid).
-
-
Loss Calculation:
-
The difference between the predicted output and the actual output is calculated using a loss function (e.g., mean squared error for regression, cross-entropy for classification).
-
-
Backpropagation:
-
The gradient of the loss function with respect to each weight is computed using the chain rule.
-
Gradients are propagated backward through the network to update the weights.
-
-
Weight Update:
-
Weights are updated using an optimization algorithm like gradient descent.
-
Formula: New Weight = Old Weight – Learning Rate * Gradient.
-
Example: In image classification, a convolutional neural network (CNN) learns to detect edges, shapes, and objects by adjusting weights during training.
Pro Tip: Use techniques like batch normalization and dropout to improve training stability and prevent overfitting.
10. What is the difference between bagging and boosting?
-
Bagging (Bootstrap Aggregating):
-
Trains multiple models independently on random subsets of the data.
-
Combines predictions by averaging (regression) or voting (classification).
-
Reduces variance and avoids overfitting.
-
Example: Random Forests.
-
-
Boosting:
-
Trains models sequentially, with each model focusing on the errors of the previous one.
-
Combines predictions using weighted averages.
-
Reduces bias and often achieves higher accuracy.
-
Example: Gradient Boosting Machines (GBM), AdaBoost.
-
Key Differences:
-
Bagging is parallel (models are independent), while boosting is sequential.
-
Bagging reduces variance, while boosting reduces bias.
-
Bagging is less prone to overfitting, while boosting requires careful tuning to avoid overfitting.
Example: Use bagging for robust, general-purpose models and boosting for high-performance models with more tuning.
Section 3: Coding and Implementation
11. Write Python code to implement linear regression from scratch.
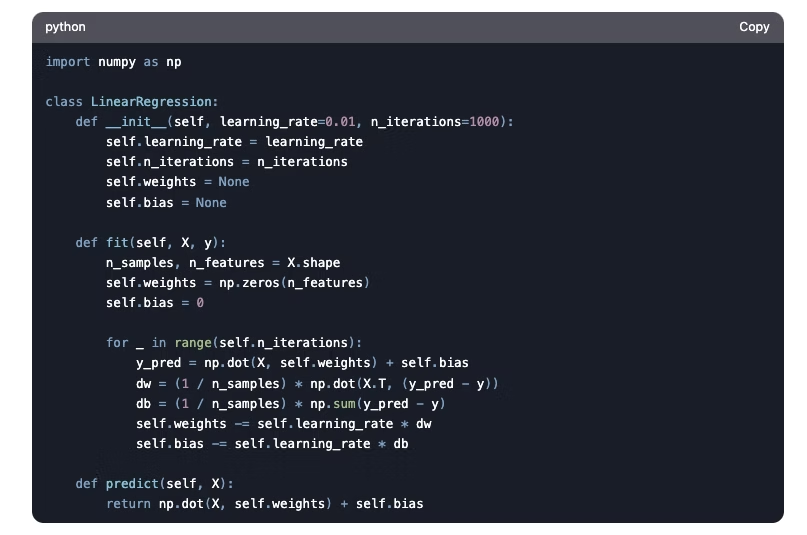
Explanation:
-
The fit method trains the model using gradient descent.
-
The predict method uses the learned weights and bias to make predictions.
-
The learning rate controls the step size during weight updates.
Pro Tip: For large datasets, use stochastic gradient descent (SGD) or mini-batch gradient descent for faster convergence.
12. How would you optimize a machine learning algorithm for large datasets?
-
Use Efficient Algorithms:
-
Replace batch gradient descent with stochastic gradient descent (SGD) or mini-batch gradient descent.
-
Use algorithms like L-BFGS or Adam for faster convergence.
-
-
Parallelize Computations:
-
Use distributed computing frameworks like Apache Spark or Dask.
-
Leverage GPUs for deep learning models.
-
-
Optimize Data Storage:
-
Store data in columnar formats like Parquet or ORC for faster retrieval.
-
Use databases like SQL or NoSQL for efficient querying.
-
-
Feature Engineering:
-
Reduce the number of features using techniques like PCA or feature selection.
-
Use dimensionality reduction to handle high-dimensional data.
-
Example: If you’re training a deep learning model on millions of images, use a GPU and mini-batch gradient descent to speed up training.
13. Write a function to calculate the precision and recall of a classification model.
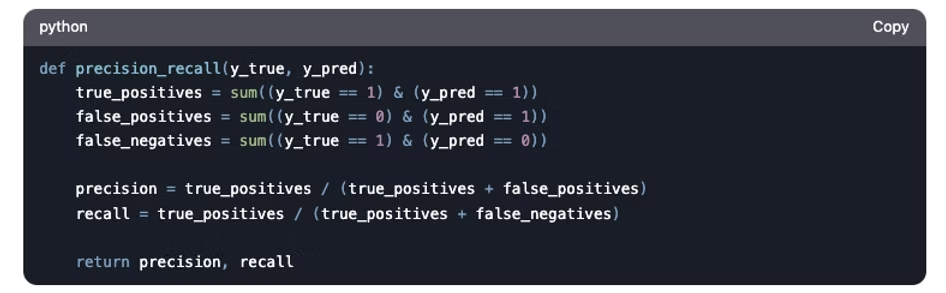
Explanation:
-
Precision measures the accuracy of positive predictions.
-
Recall measures the fraction of actual positives correctly identified.
-
Both metrics are important for imbalanced datasets.
Example: In a medical diagnosis system, recall is critical because missing a positive case (false negative) can have serious consequences.
14. How do you handle missing data in a dataset?
-
Remove Missing Data:
-
Drop rows or columns with missing values if the dataset is large enough.
-
Example: df.dropna() in pandas.
-
-
Impute Missing Data:
-
Replace missing values with the mean, median, or mode of the column.
-
Example: df.fillna(df.mean()) in pandas.
-
-
Advanced Techniques:
-
Use K-nearest neighbors (KNN) imputation to estimate missing values based on similar rows.
-
Use predictive modeling (e.g., regression) to predict missing values.
-
Example: If a dataset has missing age values, you can impute them using the median age of similar individuals.
15. Implement k-means clustering in Python.
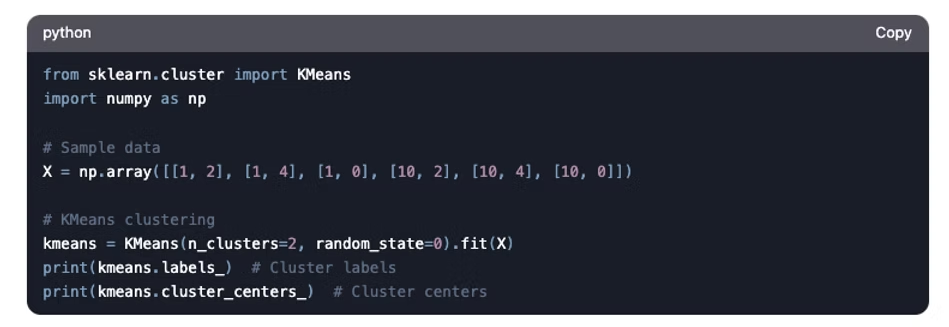
Explanation:
-
K-means groups data into k clusters by minimizing the sum of squared distances between points and their cluster centroids.
-
The algorithm iteratively updates cluster centroids and reassigns points to the nearest centroid.
Pro Tip: Use the elbow method to determine the optimal number of clusters (k).
Section 4: System Design for ML
16. How would you design a recommendation system for Amazon?
A recommendation system is critical for Amazon to personalize user experiences and drive sales. Here’s how you can design one:
Step 1: Data Collection
-
Collect user data: browsing history, purchase history, ratings, and reviews.
-
Collect product data: categories, descriptions, and metadata.
Step 2: Choose the Approach
-
Collaborative Filtering: Recommend products based on user behavior (e.g., “Users who bought this also bought that”).
-
Example: Matrix factorization techniques like Singular Value Decomposition (SVD).
-
-
Content-Based Filtering: Recommend products similar to those a user has liked.
-
Example: Use product descriptions and metadata to compute similarity scores.
-
-
Hybrid Approach: Combine collaborative and content-based filtering for better accuracy.
Step 3: Model Training
-
Use algorithms like Alternating Least Squares (ALS) for collaborative filtering.
-
Train the model on historical data to predict user preferences.
Step 4: Deployment
-
Deploy the model on AWS using services like SageMaker for scalability.
-
Use real-time data pipelines (e.g., Apache Kafka) to update recommendations dynamically.
Step 5: Evaluation
-
Measure performance using metrics like precision, recall, and mean average precision (MAP).
-
Conduct A/B testing to compare the new system with the existing one.
Example: Netflix uses a hybrid recommendation system to suggest movies and shows based on user behavior and content similarity.
17. Explain how you would deploy a machine learning model at scale.
Deploying an ML model at scale involves several steps to ensure reliability, scalability, and performance.
Step 1: Model Packaging
-
Use containerization tools like Docker to package the model and its dependencies.
-
Example: Create a Docker image with Python, TensorFlow, and your model.
Step 2: Deployment Platform
-
Use cloud platforms like AWS SageMaker, Google Cloud AI, or Azure ML.
-
Example: Deploy the model as an API endpoint using AWS SageMaker.
Step 3: API Development
-
Create RESTful APIs using frameworks like Flask or FastAPI.
-
Example: Expose a /predict endpoint that accepts input data and returns predictions.
Step 4: Scalability
-
Use load balancers and auto-scaling to handle high traffic.
-
Example: Deploy the API on Kubernetes for orchestration and scaling.
Step 5: Monitoring
-
Monitor performance using tools like Prometheus and Grafana.
-
Set up alerts for issues like high latency or low accuracy.
Example: Uber uses ML models to predict ride demand and deploys them at scale using Kubernetes and cloud platforms.
18. How do you handle data pipelines for real-time ML systems?
Real-time ML systems require efficient data pipelines to process and deliver data quickly.
Step 1: Data Ingestion
-
Use streaming platforms like Apache Kafka or AWS Kinesis to ingest real-time data.
-
Example: Ingest user clickstream data for real-time recommendations.
Step 2: Data Processing
-
Use stream processing frameworks like Apache Spark Streaming or Flink.
-
Example: Process incoming data to compute features for the ML model.
Step 3: Data Storage
-
Store processed data in a NoSQL database like Cassandra or MongoDB for quick retrieval.
-
Example: Store user preferences and product metadata for real-time lookups.
Step 4: Model Serving
-
Use a real-time serving system like TensorFlow Serving or RedisAI.
-
Example: Serve predictions for real-time fraud detection.
Step 5: Monitoring
-
Monitor the pipeline for latency, throughput, and errors.
-
Example: Use tools like Datadog or Splunk for real-time monitoring.
Example: Twitter uses real-time data pipelines to process tweets and serve personalized content.
19. What is A/B testing, and how would you use it to evaluate an ML model?
A/B testing is a statistical method to compare two versions of a model or system to determine which performs better.
Step 1: Define the Hypothesis
-
Example: “Model B will increase click-through rates (CTR) compared to Model A.”
Step 2: Split the Users
-
Randomly divide users into two groups: Group A (control) and Group B (treatment).
-
Example: Group A sees recommendations from Model A, and Group B sees recommendations from Model B.
Step 3: Run the Experiment
-
Collect data on key metrics like CTR, conversion rate, or revenue.
-
Example: Run the experiment for two weeks to gather sufficient data.
Step 4: Analyze the Results
-
Use statistical tests (e.g., t-test) to determine if the difference in performance is significant.
-
Example: If Model B has a statistically higher CTR, deploy it to all users.
Pro Tip: Ensure the sample size is large enough to detect meaningful differences.
20. How would you design a fraud detection system?
Fraud detection systems use ML to identify suspicious activities in real-time.
Step 1: Data Collection
-
Collect transaction data: amount, location, time, and user behavior.
-
Collect historical fraud data for labeling.
Step 2: Feature Engineering
-
Create features like transaction frequency, average transaction amount, and deviation from normal behavior.
-
Example: Flag transactions that are significantly larger than the user’s average.
Step 3: Model Training
-
Use algorithms like logistic regression, random forests, or neural networks.
-
Train the model on labeled data to classify transactions as fraudulent or not.
Step 4: Real-Time Detection
-
Deploy the model as a real-time API to analyze incoming transactions.
-
Example: Use AWS Lambda for serverless real-time processing.
Step 5: Feedback Loop
-
Continuously update the model with new data to improve accuracy.
-
Example: Use user feedback to refine fraud detection rules.
Example: PayPal uses ML models to detect fraudulent transactions in real-time.
Section 5: Behavioral and Leadership Principles
21. Tell me about a time you faced a challenging technical problem and how you solved it.
-
Example: “I once worked on a project where the model’s accuracy was low due to imbalanced data. I solved it by using techniques like SMOTE and adjusting class weights, which improved the model’s performance.”
-
Key Takeaways: Highlight problem-solving skills, technical expertise, and persistence.
22. How do you prioritize tasks when working on multiple projects?
-
Example: “I use the Eisenhower Matrix to categorize tasks based on urgency and importance. I also communicate with stakeholders to align on priorities.”
-
Key Takeaways: Show organizational skills, time management, and collaboration.
23. Describe a situation where you had to explain a complex ML concept to a non-technical stakeholder.
-
Example: “I explained the concept of neural networks to a marketing team by comparing it to how the human brain processes information. I used simple analogies and visuals to make it relatable.”
-
Key Takeaways: Demonstrate communication skills and the ability to simplify complex ideas.
24. Tell me about a time you disagreed with a teammate. How did you resolve it?
-
Example: “I once disagreed with a teammate on the choice of algorithm for a project. We resolved it by discussing the pros and cons of each option and running experiments to compare their performance.”
-
Key Takeaways: Show teamwork, conflict resolution, and data-driven decision-making.
25. How do you stay updated with the latest advancements in machine learning?
-
Example: “I regularly read research papers on arXiv, follow ML blogs like Towards Data Science, and participate in online courses and webinars.”
-
Key Takeaways: Highlight a commitment to continuous learning and staying current in the field.
5. Tips to Ace Amazon ML Interviews
-
Master the Basics: Ensure you have a strong understanding of ML fundamentals, algorithms, and coding.
-
Practice Coding: Use platforms like LeetCode and HackerRank to practice coding problems.
-
Understand Amazon’s Leadership Principles: Be ready to provide examples that demonstrate these principles.
-
Prepare for System Design: Practice designing scalable ML systems and data pipelines.
-
Mock Interviews: Simulate real interview scenarios to build confidence and improve communication skills.
6. How InterviewNode Can Help You Prepare
At InterviewNode, we specialize in helping software engineers like you prepare for ML interviews at top companies like Amazon. Here’s how we can help:
-
Mock Interviews: Practice with experienced ML engineers who’ve been through the process.
-
Personalized Coaching: Get tailored feedback and guidance to improve your weak areas.
-
Curated Resources: Access a library of interview questions, coding problems, and system design templates.
-
Success Stories: Learn from candidates who’ve aced their Amazon ML interviews with our help.
7. Conclusion
Preparing for an Amazon ML interview can feel overwhelming, but with the right strategy and resources, you can succeed. By mastering the top 25 questions we’ve covered in this blog, you’ll be well-equipped to tackle the technical and behavioral challenges of the interview process.
Remember, preparation is key. Use the tips and resources provided here, and don’t hesitate to reach out to InterviewNode for personalized support. You’ve got this!