Introduction
The world of technology is shifting at an unprecedented pace. From chatbots revolutionizing customer service to self-driving cars redefining transportation, Machine Learning (ML) is at the heart of this transformation. As a software engineer, you’re already skilled at problem-solving and building robust systems. But here’s the big question: Are you ready to future-proof your career by transitioning to ML?
By 2025, the demand for ML expertise will reach new heights, and software engineers equipped with ML skills will find themselves at the forefront of this technological wave. This blog explores why 2025 is the ideal time to make the leap into ML, the benefits of doing so, and how InterviewNode can help you succeed in your transition.
1. The Rise of Machine Learning & Artificial Intelligence
Over the past decade, Machine Learning (ML) has evolved from a niche academic discipline into a cornerstone of modern technology, driving innovations across various industries. This transformation is evident in the widespread adoption of ML applications, from personalized recommendations on streaming platforms to advanced fraud detection systems in finance.
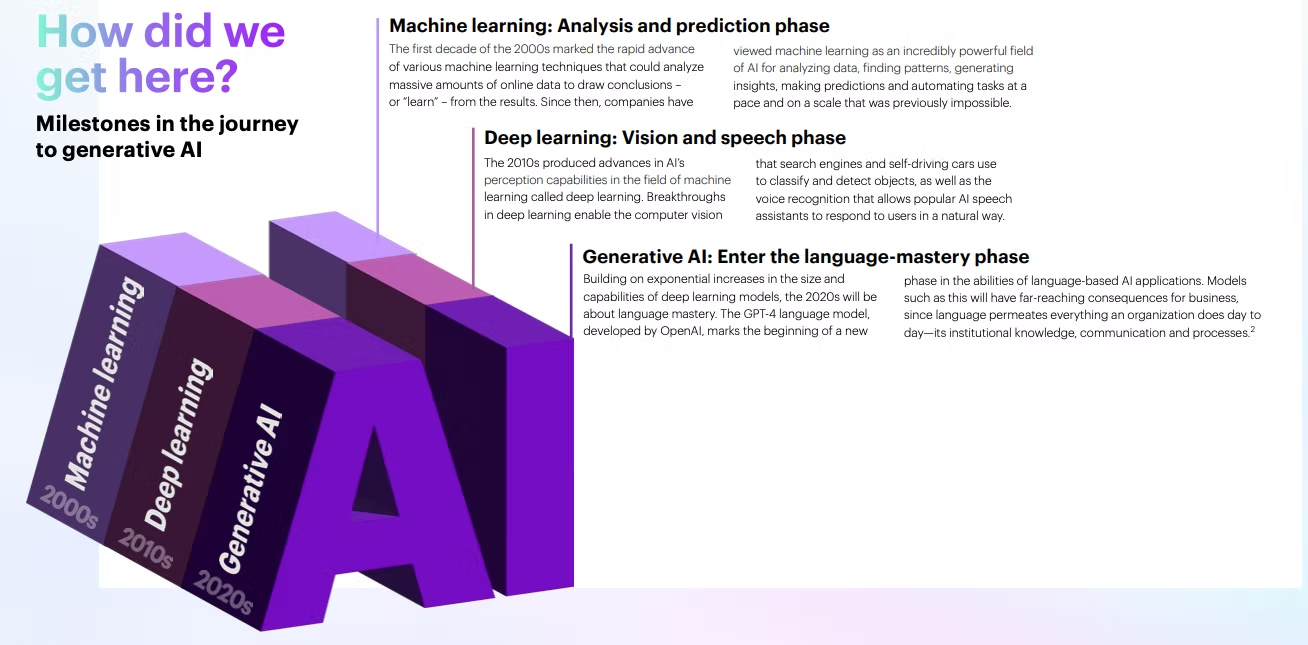
Historical Context: In the early 2010s, ML was primarily confined to research labs and specialized tech companies. However, with the advent of big data and increased computational power, ML began to permeate mainstream applications. Tech giants like Google and Amazon integrated ML into their services, setting the stage for broader industry adoption.
Current State: Today, ML is ubiquitous. Healthcare providers utilize ML algorithms for predictive diagnostics, while retailers employ them for inventory management and customer insights. The finance sector leverages ML for algorithmic trading and risk assessment. This widespread integration underscores ML’s versatility and its critical role in driving efficiency and innovation.
Future Projections: Looking ahead to 2025, the demand for ML expertise is expected to surge significantly. According to the World Economic Forum’s Future of Jobs Report 2023, roles for AI and machine learning specialists are projected to grow by 40% by 2027, adding approximately 2.6 million jobs globally.
Similarly, Analytics Insight reports that by 2025, roles such as AI specialists, data analysts, and ML engineers will dominate the job market, with an estimated 97 million new roles related to AI emerging.
This anticipated growth is driven by several factors:
-
Technological Advancements: Continuous improvements in ML algorithms and the development of more efficient computational tools are expanding the scope of ML applications.
-
Industry Adoption: Sectors such as healthcare, finance, and manufacturing are increasingly relying on ML to enhance operations, leading to a higher demand for skilled professionals.
-
Economic Impact: AI and ML are expected to contribute significantly to global GDP, with projections indicating a potential increase of up to 7% due to AI-driven productivity gains.
The Emerging Trends in AI for 2025
-
Expansion of Generative AI
As 2025 is nearing, Generative AI is set to transform creative industries by automating content creation. By 2025, it will be extensively used for producing high-quality text, images, and even video. The rise of generative AI will lead companies in marketing, advertising, and design. This shift will be owed to the increasing dependence on generative AI tools like OpenAI’s ChatGPT and DALL-E to develop personalized content. This technology will foster new forms of innovation and enhance efficiency in the creative field.
-
AI-Powered Personalization
AI will deepen its role in creating personalized experiences, particularly in sectors such as healthcare, e-commerce, and entertainment. Top entertainment conglomerates like Amazon and Netflix are already using AI algorithms to analyze consumer data and tailor recommendations. By 2025, this trend will intensify and monumentally increase its reliability on AI. This will lead AI to analyze user behaviour to provide hyper-personalized interactions and services.
-
AI in Healthcare
AI’s role in healthcare is expected to expand dramatically. From AI-powered diagnostics to robotic surgeries, the technology will revolutionize patient care. For instance, AI-driven systems like the Da Vinci Surgical System are enhancing precision in surgeries, while AI-based predictive analytics are enabling personalized medicine. Telemedicine will also benefit from AI by offering real-time diagnostics and treatment recommendations.
-
Ethical AI and Regulation
With the widespread adoption of AI, ethical considerations and regulations will become more critical. By 2025, frameworks addressing issues like data privacy, algorithmic bias, and transparency will take center stage. Governments and organizations alike will need to prioritize responsible AI practices to build trust and ensure AI benefits all society.
-
AI Augmenting Human Roles
Rather than replacing jobs, AI will increasingly augment human tasks. This will be especially prevalent in sectors such as finance, law, and education. AI will automate repetitive tasks, allowing humans to focus on more complex problem-solving and decision-making.
In summary, the trajectory of ML points towards a future where its integration into various industries will not only drive innovation but also create a substantial number of job opportunities. For software engineers, this presents a compelling case to transition into ML roles to stay at the forefront of technological advancement.
2. Why 2025 is the Ideal Time for Transition
Transitioning to Machine Learning (ML) is not just a career upgrade—it’s a strategic move to stay relevant and thrive in a rapidly evolving tech landscape. Here’s why 2025 stands out as the perfect year to make the leap:
Technological Advancements
By 2025, ML technology is expected to become more accessible and user-friendly, thanks to innovations in tools and frameworks. Key trends include:
-
Pre-trained Models and APIs: The rise of advanced pre-trained models like OpenAI’s GPT-4 and Meta’s AI platforms has already made ML more approachable for non-experts. By 2025, these tools will become even more sophisticated and widely available, allowing engineers to quickly implement ML solutions without starting from scratch.
-
Automated Machine Learning (AutoML): AutoML tools such as Google’s AutoML and Microsoft Azure ML will further streamline the process of designing, training, and deploying models, reducing the steep learning curve traditionally associated with ML.
-
Edge Computing and ML: The convergence of ML with edge computing will unlock real-time AI capabilities for IoT devices, creating demand for engineers skilled in both software development and ML.
These advancements mean that transitioning into ML will require less time and effort than ever before, making 2025 an ideal time to capitalize on this opportunity.
Unprecedented Industry Demand
The appetite for ML expertise is growing across all industries. By 2025:
-
Increased Use Cases: Industries like healthcare (predictive diagnostics), automotive (autonomous vehicles), and finance (fraud detection) will continue to expand their ML applications.
-
Shortage of Skilled Professionals: While the demand for ML experts is skyrocketing, the supply of qualified talent isn’t keeping pace. The U.S. Bureau of Labor Statistics projects a 31% growth in ML-related jobs between 2020 and 2030, a rate much faster than the average for all occupations.
This supply-demand gap creates a window of opportunity for skilled software engineers to transition and position themselves as indispensable assets.
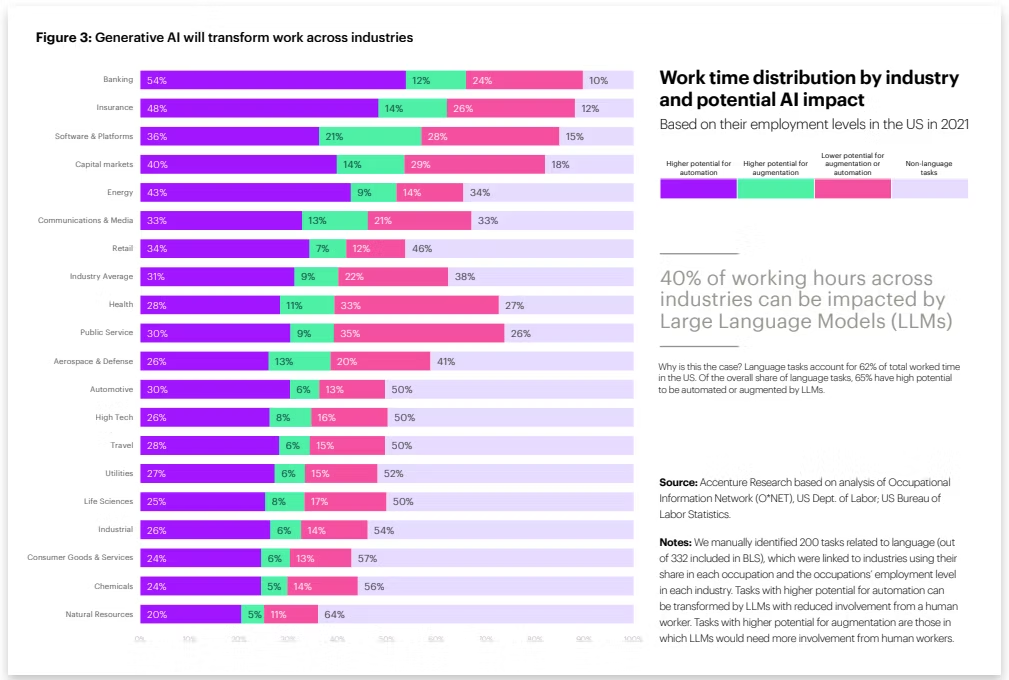
Competitive Advantage
The tech job market is becoming increasingly competitive. Here’s how transitioning to ML in 2025 gives you an edge:
-
Future-Proofing Your Career: Traditional software engineering roles are at risk of partial automation. By acquiring ML skills, you’ll stay relevant and secure in a world increasingly driven by AI and automation.
-
High-Impact Roles: ML engineers often work on projects with significant visibility and impact, such as developing AI-driven products or optimizing business processes. These roles offer a chance to influence critical decisions and innovations.
-
Cross-Functional Opportunities: ML expertise opens doors to roles that intersect with data science, product management, and strategic decision-making, broadening your career prospects.
Supportive Ecosystem for Transition
As ML gains traction, the ecosystem supporting professionals in making this transition has also matured:
-
Learning Resources: Platforms like Coursera, Udemy, and specialized ML bootcamps offer accessible and affordable ways to gain expertise.
-
Community Support: ML communities on GitHub, Kaggle, and Reddit provide collaborative environments for learning and project-building.
-
Recruitment Trends: Companies are increasingly open to hiring professionals transitioning from other tech roles, provided they demonstrate the right skills and mindset.
Economic Incentives
The financial benefits of transitioning to ML are hard to ignore:
-
Salaries: According to Glassdoor, the average ML engineer salary in the U.S. is approximately $130,000–$150,000 annually, with top-tier companies offering upwards of $200,000.
-
Growth Opportunities: Companies often provide additional perks, including stock options and bonuses, to attract and retain ML talent.
Timing is Everything
While the ML landscape is booming now, it will likely become more saturated as more professionals make the switch. By transitioning in 2025, you position yourself ahead of the curve, capturing the high-value roles before they become more competitive.
Opportunities in the ML/AI Job Market
1. AI and Machine Learning Specialists: With automation driving many changes in industries, demand for AI and machine learning (ML) specialists will continue to rise. In fact, by 2025, roles such as AI specialists, data analysts, and ML engineers will dominate the job market. This is evident from reports suggesting that 97 million new roles related to AI could emerge by 2025.
2. Data Analysts and Scientists: Data will continue to be the backbone of AI applications. As AI becomes more integrated into various processes, there will be a growing need for data analysts and scientists to interpret vast amounts of information, derive insights, and help businesses make data-driven decisions.
3. Robotics Engineers: With the rise of AI-powered robotics, engineers who can design, build, and maintain robotic systems will be in high demand. This will be especially relevant in sectors like manufacturing, healthcare, and logistics.
4. AI in Edge Computing: Edge AI, where data is processed closer to the source (e.g., in IoT devices), will offer numerous job opportunities in sectors like autonomous vehicles, smart cities, and industrial automation. Reducing latency and improving real-time decision-making will require professionals skilled in AI and edge computing.
5. AI in Sustainability: AI’s role in addressing global challenges like climate change will open up new jobs related to sustainability. AI-driven solutions in energy optimization, resource management, and carbon footprint reduction will become increasingly important, offering jobs in green technology and environmental management.
3. Benefits of Transitioning to Machine Learning
Transitioning to Machine Learning (ML) offers software engineers a wealth of benefits—ranging from personal and professional growth to financial rewards and long-term job security. Here’s a closer look at the key advantages:
1. Career Growth and Opportunities
The shift to ML opens doors to exciting new career paths and opportunities for professional advancement:
-
High-Demand Roles: ML engineers, data scientists, and AI specialists are among the most sought-after professionals in the tech industry. Companies across sectors—tech, finance, healthcare, retail, and beyond—are investing heavily in AI/ML to drive innovation and efficiency.
-
Diverse Career Paths: Transitioning to ML allows you to explore various roles, including:
-
AI/ML Engineer
-
Data Scientist
-
Research Scientist
-
AI Product Manager
-
AI Solutions Architect
-
NLP Engineer (Natural Language Processing)
-
-
Room for Advancement: In ML, there’s ample opportunity for vertical growth. Professionals often start as engineers or analysts and move up to lead roles, managerial positions, or specialized research opportunities.
2. Financial Incentives
A career in ML isn’t just rewarding intellectually—it’s also highly lucrative. Here’s why:
-
Competitive Salaries: According to data from platforms like Glassdoor and Levels.fyi, the average base salary for ML engineers in the U.S. ranges from $130,000 to $150,000 annually. Professionals at top-tier companies like Google, Meta, and Apple often earn total compensation packages exceeding $200,000.
-
Global Opportunities: ML skills are in demand worldwide. This creates opportunities to work in high-paying markets like Silicon Valley, London, Singapore, or Berlin.
-
Perks and Bonuses: Companies seeking top ML talent frequently offer additional incentives such as stock options, sign-on bonuses, and performance-based bonuses.
3. Intellectual Stimulation
ML offers an unparalleled opportunity to work on cutting-edge technology and solve challenging problems. As an ML professional, you’ll:
-
Work on Innovative Projects: From creating AI-driven recommendation systems to developing computer vision models, ML projects are often at the forefront of innovation.
-
Tackle Complex Problems: ML involves a mix of programming, data analysis, mathematics, and domain expertise, providing a constant intellectual challenge.
-
Make an Impact: ML professionals often work on projects with tangible outcomes—whether it’s improving healthcare outcomes through predictive models or optimizing supply chains for reduced waste.
4. Job Security
In a world increasingly driven by automation and data, ML expertise provides unparalleled job security:
-
Resilient to Automation: While many software engineering roles are at risk of being automated, ML roles are centered on designing and improving those very systems, making them future-proof.
-
Endless Demand: As businesses collect more data, the need for skilled professionals to analyze and extract insights from it will only grow. According to the U.S. Bureau of Labor Statistics, ML-related roles are projected to grow by over 30% in the next decade.
5. High-Impact Work
ML professionals often have the opportunity to work on projects that create significant impact, such as:
-
Healthcare: Predicting disease outbreaks, diagnosing illnesses using imaging, and personalizing treatments.
-
Environment: Improving energy efficiency, monitoring deforestation, and optimizing waste management.
-
Social Good: Creating tools to combat misinformation, improve accessibility, and enhance education.
This sense of purpose and contribution can make a career in ML incredibly fulfilling.
6. Versatility Across Industries
One of the unique advantages of ML is its applicability across a wide range of industries. As an ML professional, you can work in sectors like:
-
Healthcare: Building predictive models for early diagnosis.
-
Finance: Creating fraud detection algorithms and optimizing trading strategies.
-
Retail: Personalizing customer experiences and managing supply chains.
-
Entertainment: Designing recommendation systems for platforms like Netflix or Spotify.
-
Transportation: Developing autonomous vehicles and route optimization tools.
This versatility ensures that ML professionals have the flexibility to switch industries while maintaining high demand for their skills.
7. Opportunity for Lifelong Learning
ML is a rapidly evolving field. For those who love continuous learning, ML offers a perfect career path:
-
Constant Innovation: With advancements like generative AI, federated learning, and neural architecture search, there’s always something new to explore.
-
Access to Cutting-Edge Research: ML professionals often have access to the latest research, tools, and methodologies, keeping them at the forefront of technology.
-
Cross-Disciplinary Knowledge: ML allows you to combine expertise from multiple domains, such as neuroscience, physics, linguistics, and more, depending on the projects you work on.
8. Entrepreneurial Opportunities
For software engineers with an entrepreneurial mindset, ML presents an excellent avenue to start your own ventures. Examples include:
-
AI-Powered Startups: Developing niche AI solutions for businesses or consumers.
-
Consulting Services: Offering ML expertise to companies that lack in-house talent.
-
Open-Source Tools: Contributing to or monetizing open-source ML projects.
4. Challenges in Making the Switch
While transitioning to ML offers numerous benefits, it also comes with challenges:
-
Steep Learning Curve: Mastering ML requires a strong foundation in mathematics, statistics, and programming.
-
Real-World Experience: Many companies expect candidates to have hands-on experience with ML projects.
-
Intense Competition: The growing popularity of ML means you’ll need to stand out to secure top roles.
But don’t worry—these challenges are surmountable with the right guidance and resources.
5. Essential Skills for Machine Learning Engineers
To succeed as an ML engineer, you’ll need to build expertise in the following areas:
Programming
-
Master Python (a must-have) and consider R or Julia for statistical analysis.
Mathematics
-
Brush up on linear algebra, calculus, probability, and statistics.
ML Algorithms
-
Understand how supervised, unsupervised, and reinforcement learning algorithms work.
Data Management
-
Learn to preprocess, clean, and visualize datasets for analysis.
Frameworks
-
Gain proficiency in TensorFlow, PyTorch, scikit-learn, and other ML tools.
Soft Skills
-
Problem-solving, communication, and collaboration are crucial for working in cross-functional teams.
6. Steps to Transition from Software Engineering to Machine Learning
1. Education
Start with online courses, bootcamps, or even advanced degrees specializing in AI and ML. Platforms like Coursera, edX, and Udemy offer excellent ML programs.
2. Build a Portfolio
Work on small ML projects, such as creating a recommendation system or analyzing datasets. Host your projects on GitHub to showcase your skills.
3. Gain Practical Experience
Contribute to open-source ML projects, participate in Kaggle competitions, or seek internships to gain real-world experience.
4. Network Actively
Attend ML conferences, join online forums like Reddit’s ML community, and connect with professionals on LinkedIn.
5. Prepare for Interviews
ML interviews are often technical and require in-depth knowledge of algorithms and frameworks. Practice solving ML problems and explaining your approach clearly.
7. How InterviewNode Can Assist in Your Transition
At InterviewNode, we specialize in helping software engineers like you succeed in ML interviews. Here’s how we can make your journey smoother:
-
Customized Guidance: Tailored strategies to match your unique career goals and strengths.
-
Expert Coaching: Mock interviews and feedback from professionals who’ve worked at top tech companies.
-
Comprehensive Resources: Access to ML-specific study materials, cheat sheets, and problem-solving exercises.
-
Proven Success: Countless clients have transitioned to coveted ML roles at companies like Google, Facebook, and OpenAI with our help.
Conclusion
The world is rapidly shifting towards an AI-driven future, and Machine Learning is at the center of this transformation. For software engineers, transitioning to ML in 2025 isn’t just a smart career move—it’s a necessary step to stay relevant, competitive, and fulfilled in the tech industry.
The challenges may seem daunting, but with a clear roadmap and support from InterviewNode, you can make the leap confidently. Ready to future-proof your career and land your dream ML job? Let’s get started today!